Events
6th NLTP Content Meeting Session
🌐 Discussion Topic:
Assessing the Reliability of Annotations in the Context of LLM Predictions and Explanations
This event focused on evaluating the reliability of human annotations in NLP tasks and exploring whether Generative AI (GenAI) models can serve as viable alternatives. The research examined demographic influences on labeling decisions and tested explainable AI (XAI) techniques to improve model transparency.
🔍 Overview of the Presentation
This study highlights the importance of annotation quality in NLP model training, particularly in subjective tasks such as sexism detection. Using data from the EXIST 2024 challenge, researchers analyzed how demographic variables (gender, age, ethnicity, education, and region) influence annotation consistency. A Generalized Linear Mixed Model (GLMM) was used to quantify these effects, alongside an evaluation of GPT-4o, LLaMA 3.2, and LLaMA 3.3 models.
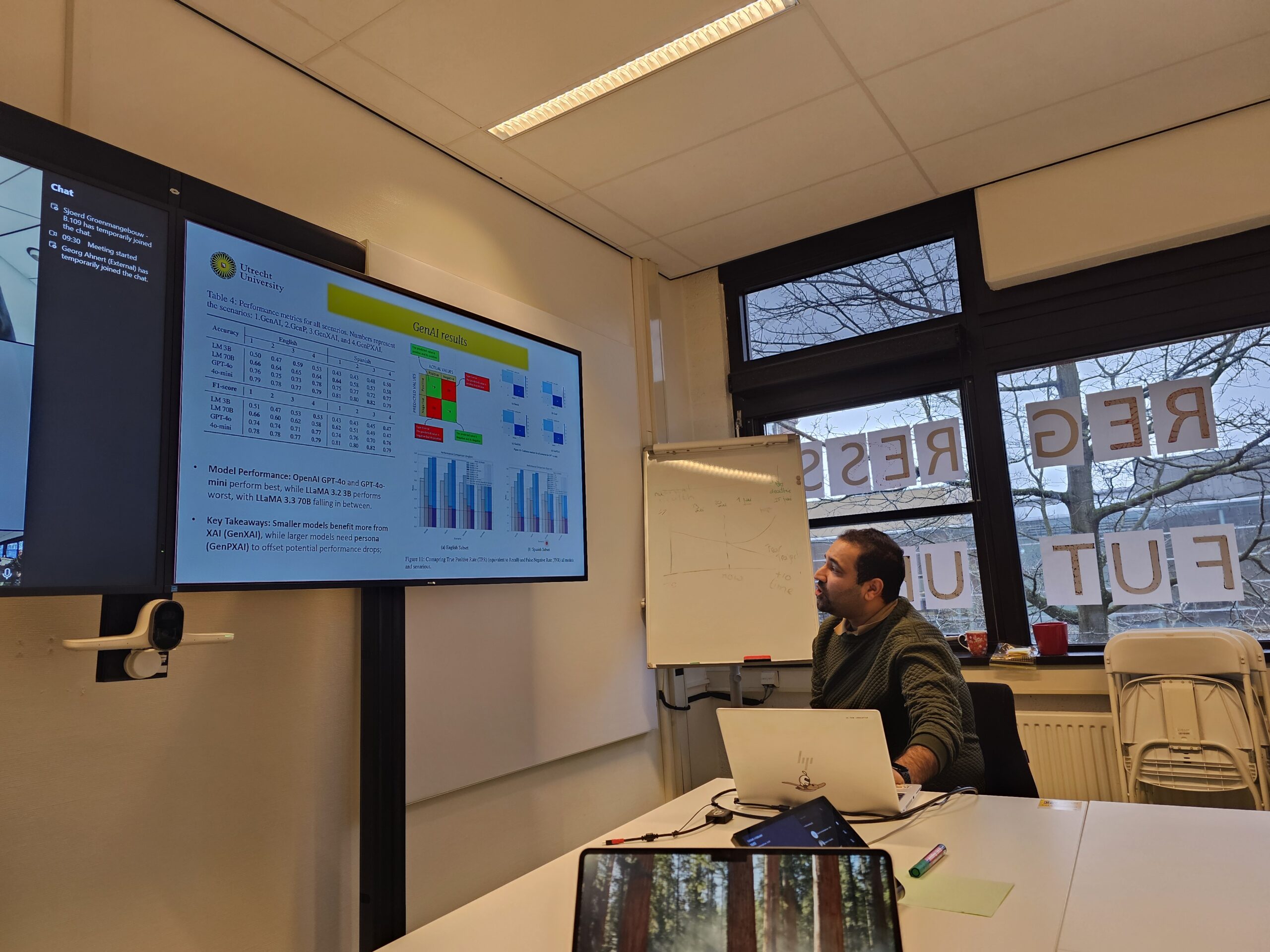
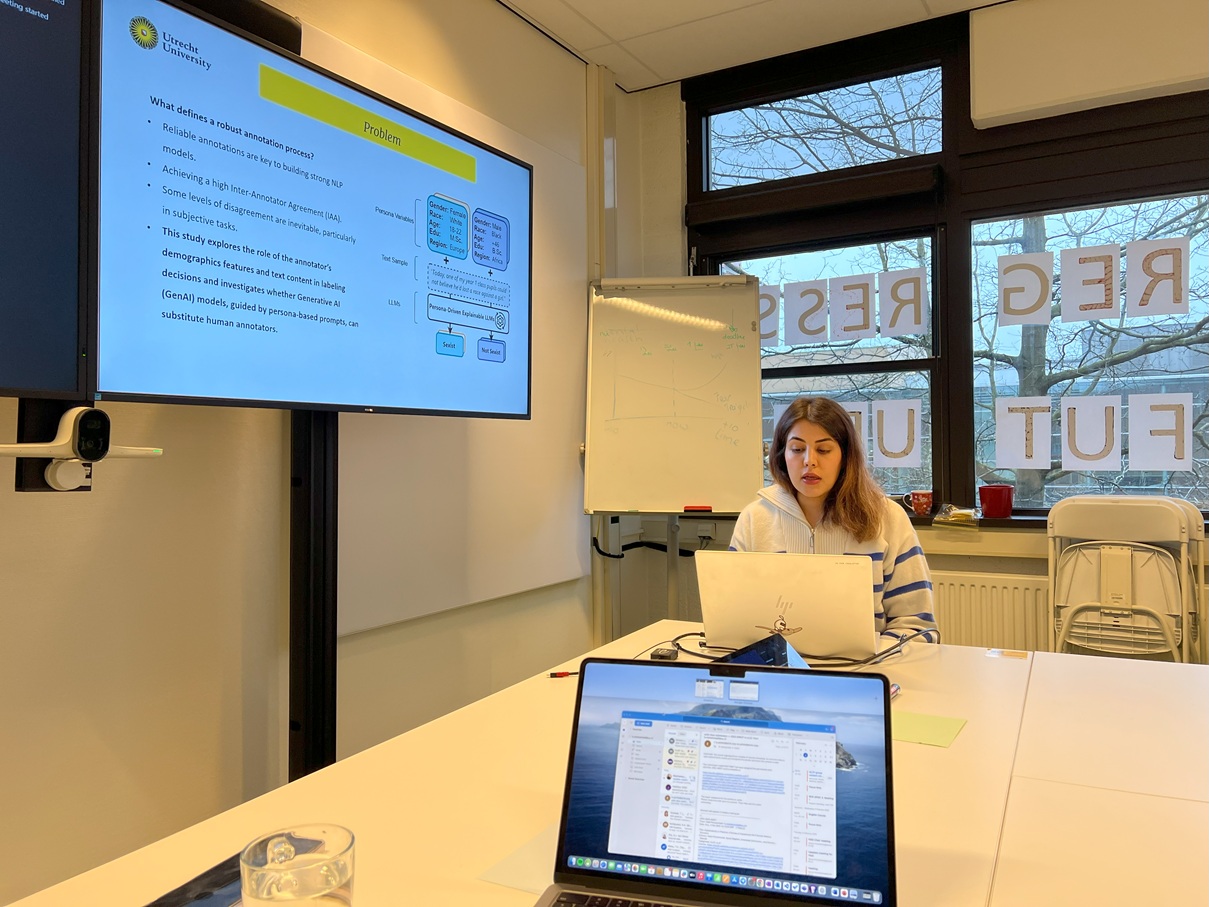
🚨 Highlights of Insights
- AI vs. Human Annotation : AI models show potential but cannot fully replace human annotators due to bias and context sensitivity.
- Demographic Influence : While tweet-specific content had the greatest impact, certain demographic groups showed distinct labeling patterns.
- Explainable AI (XAI) Techniques : SHAP values were tested to enhance model interpretability and performance.
🚀 Current Progress
✅ Analyzed annotation variability in sexism detection datasets
✅ Compared GenAI model annotations with human annotations
✅ Integrated XAI techniques to improve AI transparency
🎯 Future Goals
🔹 Expand explainability techniques for AI-assisted annotation
🔹 Increase language coverage beyond English & Spanish
🔹 Enhance fairness and reliability in AI annotation frameworks
🌟 Presenters
This insightful session was presented by Hadi Mohammadi and Tina Shahedi.